Articles & Issues
- Language
- korean
- Conflict of Interest
- In relation to this article, we declare that there is no conflict of interest.
- Publication history
-
Received March 27, 2010
Accepted April 19, 2010
-
This is an Open-Access article distributed under the terms of the Creative Commons Attribution Non-Commercial License (http://creativecommons.org/licenses/bync/3.0) which permits unrestricted non-commercial use, distribution, and reproduction in any medium, provided the original work is properly cited.
Copyright © KIChE. All rights reserved.
All issues
화상분석을 이용한 소프트 센서의 설계와 산업응용사례 2. 인조대리석의 품질 자동 분류
Soft Sensor Design Using Image Analysis and its Industrial Applications Part 2. Automatic Quality Classification of Engineered Stone Countertops
동국대학교 에너지환경시스템학부, 780-714 경북 경주시 석장동 707 1부경대학교 화학공학과, 608-739 부산광역시 남구 용당동 산 100
Department of Energy & Environmental Systems, Dongguk University, 707 Seokjang-dong, Gyeonju-si, Gyeongbuk 780-714, Korea 1Department of Chemical Engineering, Pukyong National University, San 100, Yongdang-dong, Nam-gu, Busan 608-739, Korea
Korean Chemical Engineering Research, August 2010, 48(4), 483-489(7), NONE Epub 8 September 2010
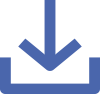
Abstract
본 연구에서는 화상분석(image analysis)에 기반한 소프트 센서를 설계하고, 이를 색상-질감 특성을 가진 제품의 외관품질 자동분류에 적용하였다. 색상과 질감(texture)을 동시에 가진 화상을 분석하기 위해 다중해상도 다변량 화상분석(Multiresolutional Multivariate Image Analysis, MR-MIA) 기법을 이용하였으며, 자동 분류를 위한 감독 학습법(supervised learning)으로는 Fisher의 판별분석(Fisher’s discriminant analysis)을 사용하였다. 잠재변수법의 하나인 Fisher의 판별분석을 사용하였기 때문에, 제품의 외관을 서로 다른 불연속적인 부류로의 분류할 수 있을 뿐 아니라, 연속적인 외관 변화를 일관적이고 정량적으로 추정함은 물론, 외관의 특성 해석 또한 가능하였다. 이 방법은 인조대리석 제조 공정에서 중간 및 최종 제품의 외관 품질을 자동으로 분류하는 데에 성공적으로 적용되었다.
An image analysis-based soft sensor is designed and applied to automatic quality classification of product appearance with color-textural characteristics. In this work, multiresolutional multivariate image analysis (MR-MIA) is used in order to analyze product images with color as well as texture. Fisher’s discriminant analysis (FDA) is also used as a supervised learning method for automatic classification. The use of FDA, one of latent variable methods, enables us not only to classify products appearance into distinct classes, but also to numerically and consistently estimate product appearance with continuous variations and to analyze characteristics of appearance. This approach is successfully applied to automatic quality classification of intermediate and final products in industrial manufacturing of engineered stone countertops.
Keywords
References
Holtham PN, Nguyen KK, Int. J. Miner. Process., 64, 163 (2002)
Kaartinen J, Hotonen J, Hyotyniemi H, Miettunen J, Control Eng. Practice, 14, 1455 (2006)
Liu JJ, Korean Chem. Eng. Res., 48(4), 475 (2010)
Kirn D, Han C, Liu JJ, Ind. Eng. Chem. Res., 48(5), 2590 (2009)
Liu JJ, Macgregor JF, Ind. Eng. Chem. Res., 44(13), 4687 (2005)
Liu JJ, MacGregor JF, Chemometrics Intell. Lab. Syst., 85, 119 (2007)
Fisher RA, Annals of Eugenics, 7, 179 (1936)
Geladi P, Grahn H, Multivariate Image Analysis, John Wiley & Sons, Chichester, UK (1996)
Mallat SG, IEEE Transactions on Pattern Analysis and Machine Intelligence, 11, 674 (1989)
Bharati M, MacGregor JF, Champagne M, TAPPI J., 3, 8 (2004)
Yu HL, MacGregor JF, AIChE J., 50(7), 1474 (2004)
Pereira AC, Reis MS, Saraiva PM, Ind. Eng. Chem. Res., 48(2), 988 (2009)
Bharati M, Liu JJ, MacGregor JF, Chemometrics and Intelligent Laboratory Systems, 72, 57 (2004)
Geladi P, Chemometrics and Intelligent Laboratory Systems, 14, 209 (1992)
Lied TT, Geladi P, Esbensen K, J. Chemometrics, 14, 585 (2000)
Vetterli M, Kovacevic J, Wavelets and Subband Coding, Prentice Hall, Englewood Cliffs (1995)
Duda RO, Hart PE, Stork DG, Pattern Classification. 2nd ed., Wiley-Interscience, New York (2001)
Demirkol A, Demir Z, Emre EA, Journal of Information Science and Engineering, 21, 819 (2005)
Van de Wouwer G, Wavelets for Multiscale Texture Analysis, Ph.D Thesis, University of Antwerp, Antwerp, Belgium (1998)
Kaartinen J, Hotonen J, Hyotyniemi H, Miettunen J, Control Eng. Practice, 14, 1455 (2006)
Liu JJ, Korean Chem. Eng. Res., 48(4), 475 (2010)
Kirn D, Han C, Liu JJ, Ind. Eng. Chem. Res., 48(5), 2590 (2009)
Liu JJ, Macgregor JF, Ind. Eng. Chem. Res., 44(13), 4687 (2005)
Liu JJ, MacGregor JF, Chemometrics Intell. Lab. Syst., 85, 119 (2007)
Fisher RA, Annals of Eugenics, 7, 179 (1936)
Geladi P, Grahn H, Multivariate Image Analysis, John Wiley & Sons, Chichester, UK (1996)
Mallat SG, IEEE Transactions on Pattern Analysis and Machine Intelligence, 11, 674 (1989)
Bharati M, MacGregor JF, Champagne M, TAPPI J., 3, 8 (2004)
Yu HL, MacGregor JF, AIChE J., 50(7), 1474 (2004)
Pereira AC, Reis MS, Saraiva PM, Ind. Eng. Chem. Res., 48(2), 988 (2009)
Bharati M, Liu JJ, MacGregor JF, Chemometrics and Intelligent Laboratory Systems, 72, 57 (2004)
Geladi P, Chemometrics and Intelligent Laboratory Systems, 14, 209 (1992)
Lied TT, Geladi P, Esbensen K, J. Chemometrics, 14, 585 (2000)
Vetterli M, Kovacevic J, Wavelets and Subband Coding, Prentice Hall, Englewood Cliffs (1995)
Duda RO, Hart PE, Stork DG, Pattern Classification. 2nd ed., Wiley-Interscience, New York (2001)
Demirkol A, Demir Z, Emre EA, Journal of Information Science and Engineering, 21, 819 (2005)
Van de Wouwer G, Wavelets for Multiscale Texture Analysis, Ph.D Thesis, University of Antwerp, Antwerp, Belgium (1998)