Articles & Issues
- Language
- korean
- Conflict of Interest
- In relation to this article, we declare that there is no conflict of interest.
- Publication history
-
Received April 29, 2020
Accepted June 11, 2020
-
This is an Open-Access article distributed under the terms of the Creative Commons Attribution Non-Commercial License (http://creativecommons.org/licenses/bync/3.0) which permits unrestricted non-commercial use, distribution, and reproduction in any medium, provided the original work is properly cited.
Copyright © KIChE. All rights reserved.
All issues
인공지능 기반 질소산화물 배출량 예측을 위한 연구모형 개발
Development of Prediction Model for Nitrogen Oxides Emission Using Artificial Intelligence
포항공과대학교 화학공학과, 37673 경상북도 포항시 남구 청암로 77
Department of Chemical Engineering, Pohang University of Science and Technology, 77, Cheongam-ro, Nam-gu, Pohang-si, Gyeongsangbuk-do, 37673, Korea
Korean Chemical Engineering Research, November 2020, 58(4), 588-595(8), 10.9713/kcer.2020.58.4.588 Epub 29 October 2020
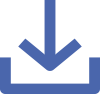
Abstract
지속적으로 강화되는 환경오염 물질 배출 규제로 인해, 질소 산화물(NOx)의 배출량 예측 및 관리는 산업 현장에서 많은 관심을 받고 있다. 본 연구에서는 인공지능 기반 질소산화물 배출량 예측모델 개발을 위한 연구모형을 제안하였다. 제안된 연구모형은 데이터의 전처리 과정부터 인공지능 모델의 학습 및 평가까지 모두 포함하고 있으며, 시계열 특성을 가지는 NOx 배출량을 예측하기 위하여 순환 신경망 중 하나인 Long Short-Term Memory (LSTM) 모델을 활용하였다. 또한 의사결정나무 기법을 활용하여 LSTM의 time window를 모델 학습 이전에 선정하는 방법을 채택하였다. 본 연구에서 제안된 연구모형의 NOx 배출량 예측 모델은 가열로에서 확보한 조업 데이터로 학습되었으며, 최적 모델은 hyper-parameter를 조절하여 개발되었다. 개발된 LSTM 모델은 학습 데이터 및 평가 데이터에 대하여 모두 93% 이상의 NOx 배출량 예측 정확도를 나타내었다. 본 연구에 제안된 연구모형은 시계열 특성을 가지는 다양한 대기오염 물질의 배출량 예측모델 개발에 응용될 수 있을 것으로 기대된다.
Prediction and control of nitrogen oxides (NOx) emission is of great interest in industry due to stricter environmental regulations. Herein, we propose an artificial intelligence (AI)-based framework for prediction of NOx emission. The framework includes pre-processing of data for training of neural networks and evaluation of the AI-based models. In this work, Long-Short-Term Memory (LSTM), one of the recurrent neural networks, was adopted to reflect the time series characteristics of NOx emissions. A decision tree was used to determine a time window of LSTM prior to training of the network. The neural network was trained with operational data from a heating furnace. The optimal model was obtained by optimizing hyper-parameters. The LSTM model provided a reliable prediction of NOx emission for both training and test data, showing an accuracy of 93% or more. The application of the proposed AI-based framework will provide new opportunities for predicting the emission of various air pollutants with time series characteristics.
References
Studzinski W, Liiva P, Choate P, Acker W, SAE Tech. Pap., 932815(1993).
Lee H, National Assembly Research Service, 36 (2019).
Karim ZAA, Khan MY, Rashid A, Aziz A, Hagos FY, Platform : A Journal of Engineering, 3, 1-21(2019).
Xu M, Azevedo JLT, Carvalho MG, Fuel, 79, 1611 (2000)
Khoshhal A, Rahimi M, Alsairafi AA, Int. Commun. Heat Mass Transfer, 38, 1421 (2011)
Ferretti G, Piroddi L, J. Eng. Gas Turbines Power, 123, 465 (2001)
Reinbacher F, Regele JD, Combust. Theor. Model., 22, 110 (2018)
Joo S, Yoon J, Kim J, Lee M, Yoon Y, Appl. Therm. Eng., 80, 436 (2015)
Park C, Kim Y, J. Korean Soc. Qual. Manag., 46, 739 (2018)
Taghavifar H, Taghavifar H, Mardani A, Mohebbi A, Fuel, 125, 81 (2014)
Tabachnick J, Fidell BG, Ullman LS, Using Multivariate Statistics, Pearson Education, London (2007).
Meyler A, Kenny G, Quinn T, Munich Personal RePEc Archive, 11359 (1998)
Khashei M, Bijari M, Raissi Ardali GA, Neurocomputing, 72(4-6), 956 (2009)
Zhang G, Patuwo BE, Hu MY, Int. J. Forecast., 14(1), 35 (1998)
Song X, Liu Y, Xue L, Wang J, Zhang J, Wang J, Jiang L, Cheng Z, J. Petrol. Sci. Eng., 186, 106682 (2020)
Li Y, Cao H, Procedia Comput. Sci., 129, 277 (2018)
Karakoyun ES, Cıbıkdiken AO, Proceedings of the Multidisciplinary Academic Conference, 171-179(2018).
Junninen H, Niska H, Tuppurainen K, Ruuskanen J, Kolehmainen M, Atmos. Environ., 38, 2895 (2004)
Sola J, Sevilla J, IEEE Trans. Nucl. Sci., 44, 1464 (1997)
Benesty J, Chen J, Huang Y, Cohen I, Noise Reduction in Speech Processing, Springer-Verlag Berlin Heidelberg(2009).
Kuhn M, J. Stat. Softw., 28, 1 (2008)
Kwon SH, Lee J, Chung G, J. Korean Soc. Hazard Mitig., 17, 315 (2017)
Dong C, Jin B, Li D, Waste Manage., 23, 103 (2003)
Taghavifar H, Taghavifar H, Mardani A, Mohebbi A, Khalilarya S, Jafarmadar S, J. Clean Prod., 112, 1729 (2016)
Chattopadhyay S, Acta Geophys., 55, 369 (2007)
Connor JT, Martin RD, Atlas LE, EEE Trans. Neural Networks, 5, 240 (1994)
Hochreiter S, Neural Comput., 1780, 1735 (1997)
Olah C, Retrieved from http://colah.github.io/posts/2015-08-Understanding-LSTMs/.
Freeman BS, Taylor G, Gharabaghi B, The J, J. Air Waste Manage. Assoc., 68, 866 (2018)
Selvin S, Vinayakumar R, Gopalakrishnan EA, Menon VK, Soman KP, 2017 International Conference on Advances in Computing, Communications and Informatics (ICACCI), 1643-1647(2017).
Safavian SR, Landgrebe D, IEEE Trans. Syst. Man. Cybern, 21, 660 (1991)
Lee H, National Assembly Research Service, 36 (2019).
Karim ZAA, Khan MY, Rashid A, Aziz A, Hagos FY, Platform : A Journal of Engineering, 3, 1-21(2019).
Xu M, Azevedo JLT, Carvalho MG, Fuel, 79, 1611 (2000)
Khoshhal A, Rahimi M, Alsairafi AA, Int. Commun. Heat Mass Transfer, 38, 1421 (2011)
Ferretti G, Piroddi L, J. Eng. Gas Turbines Power, 123, 465 (2001)
Reinbacher F, Regele JD, Combust. Theor. Model., 22, 110 (2018)
Joo S, Yoon J, Kim J, Lee M, Yoon Y, Appl. Therm. Eng., 80, 436 (2015)
Park C, Kim Y, J. Korean Soc. Qual. Manag., 46, 739 (2018)
Taghavifar H, Taghavifar H, Mardani A, Mohebbi A, Fuel, 125, 81 (2014)
Tabachnick J, Fidell BG, Ullman LS, Using Multivariate Statistics, Pearson Education, London (2007).
Meyler A, Kenny G, Quinn T, Munich Personal RePEc Archive, 11359 (1998)
Khashei M, Bijari M, Raissi Ardali GA, Neurocomputing, 72(4-6), 956 (2009)
Zhang G, Patuwo BE, Hu MY, Int. J. Forecast., 14(1), 35 (1998)
Song X, Liu Y, Xue L, Wang J, Zhang J, Wang J, Jiang L, Cheng Z, J. Petrol. Sci. Eng., 186, 106682 (2020)
Li Y, Cao H, Procedia Comput. Sci., 129, 277 (2018)
Karakoyun ES, Cıbıkdiken AO, Proceedings of the Multidisciplinary Academic Conference, 171-179(2018).
Junninen H, Niska H, Tuppurainen K, Ruuskanen J, Kolehmainen M, Atmos. Environ., 38, 2895 (2004)
Sola J, Sevilla J, IEEE Trans. Nucl. Sci., 44, 1464 (1997)
Benesty J, Chen J, Huang Y, Cohen I, Noise Reduction in Speech Processing, Springer-Verlag Berlin Heidelberg(2009).
Kuhn M, J. Stat. Softw., 28, 1 (2008)
Kwon SH, Lee J, Chung G, J. Korean Soc. Hazard Mitig., 17, 315 (2017)
Dong C, Jin B, Li D, Waste Manage., 23, 103 (2003)
Taghavifar H, Taghavifar H, Mardani A, Mohebbi A, Khalilarya S, Jafarmadar S, J. Clean Prod., 112, 1729 (2016)
Chattopadhyay S, Acta Geophys., 55, 369 (2007)
Connor JT, Martin RD, Atlas LE, EEE Trans. Neural Networks, 5, 240 (1994)
Hochreiter S, Neural Comput., 1780, 1735 (1997)
Olah C, Retrieved from http://colah.github.io/posts/2015-08-Understanding-LSTMs/.
Freeman BS, Taylor G, Gharabaghi B, The J, J. Air Waste Manage. Assoc., 68, 866 (2018)
Selvin S, Vinayakumar R, Gopalakrishnan EA, Menon VK, Soman KP, 2017 International Conference on Advances in Computing, Communications and Informatics (ICACCI), 1643-1647(2017).
Safavian SR, Landgrebe D, IEEE Trans. Syst. Man. Cybern, 21, 660 (1991)