Articles & Issues
- Language
- korean
- Conflict of Interest
- In relation to this article, we declare that there is no conflict of interest.
- Publication history
-
Received December 29, 2020
Accepted February 23, 2021
-
This is an Open-Access article distributed under the terms of the Creative Commons Attribution Non-Commercial License (http://creativecommons.org/licenses/bync/3.0) which permits unrestricted non-commercial use, distribution, and reproduction in any medium, provided the original work is properly cited.
Copyright © KIChE. All rights reserved.
All issues
해수 이용 LNG 재기화 공정의 딥러닝과 AutoML을 이용한 동적모델링
AutoML and Artificial Neural Network Modeling of Process Dynamics of LNG Regasification Using Seawater
1명지대학교 화학공학과, 17058 경기도 용인시 처인구 명지로 116 2명지대학교 재난안전학과, 17058 경기도 용인시 처인구 명지로 116 3Department of Disaster and Safety Myongji University, Yongin, Gyeonggi-do, 17058, Korea
1Department of Chemical Engineering, Myongji University, Yongin, Gyeonggi-do, 17058, Korea 2Department of Disaster and Safety, Myongji University, Yongin, Gyeonggi-do, 17058, Korea 3명지대학교, 재난안전학과, 17058 경기도 용인시 처인구 명지로 116, Korea
dongil@mju.ac.kr
Korean Chemical Engineering Research, May 2021, 59(2), 209-218(10), 10.9713/kcer.2021.59.2.209 Epub 3 May 2021
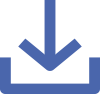
Abstract
ORV의 열교환 효율 향상 및 운전 최적화를 위한, first principle 기반 모델링 연구들이 수행되어왔지만, ORV의 열전달 계수는 시간, 위치에 따라 불규칙한 시스템으로, 복잡한 모델링 과정을 거친다. 본 연구는 복잡한 시스템에 대한 데이터 기반 모델링의 실효성을 확인하고자, LNG 재기화 공정의 실제 운전데이터를 이용해, ORV의 해수 유량, 해수온도, LNG 유량 변화에 따른 토출 NG 온도 및 토출 해수 온도의 동적 변화 예측이 가능한, FNN, LSTM 및 AutoML 기반 모델링을 진행하였다. 예측 정확도는 MSE 기준 LSTM > AutoML > FNN 순으로 좋은 성능을 보였다. 기계학습 모델의 자동설계 방법인 AutoML의 성능은 개발된 FNN보다 뛰어났으며, 모델 개발 전체소요시간은 복잡한 모델인 LSTM 대비 1/15로 크게 차이를 보여 AutoML의 활용 가능성을 보였다. LSTM과 AutoML을 이용한 토출 NG 및 토출 해수 온도의 예측은 0.5 K 미만의 오차를 보였다. 예측모델을 활용해, 겨울철 ORV를 이용해 처리 가능한 LNG 기화량의 실시간 최적화를 수행하여, 기존 대비 최대 23.5%의 LNG를 추가 처리 가능함을 확인하였고, 개발된 동적 예측모델 기반의 ORV 최적 운전 가이드라인을 제시하였다.
First principle-based modeling studies have been performed to improve the heat exchange efficiency of ORV and optimize operation, but the heat transfer coefficient of ORV is an irregular system according to time and location, and it undergoes a complex modeling process. In this study, FNN, LSTM, and AutoML-based modeling were performed to confirm the effectiveness of data-based modeling for complex systems. The prediction accuracy indicated high performance in the order of LSTM > AutoML > FNN in MSE. The performance of AutoML, an automatic design method for machine learning models, was superior to developed FNN, and the total time required for model development was 1/15 compared to LSTM, showing the possibility of using AutoML. The prediction of NG and seawater discharged temperatures using LSTM and AutoML showed an error of less than 0.5K. Using the predictive model, real-time optimization of the amount of LNG vaporized that can be processed using ORV in winter is performed, confirming that up to 23.5% of LNG can be additionally processed, and an ORV optimal operation guideline based on the developed dynamic prediction model was presented.
References
Mokhatab S, Mak JY, Valappil JV, Wood DA, Gulf Professional Publishing, 90 (2014).
Su H, Yu S, Fan J, Ling X, J. Comput., 9(2) (2014)
Wang M, Jin T, Tang K, Chen G, Natural Gas Industry, 33, 102 (2013)
Jin T, Wang M, Tang K, Cryogenics, 61, 127 (2014)
Deng Z, Hui K, Zhang Y, Cao Y, Appl. Therm. Eng., 106, 721 (2016)
Cheng H, Ju Y, Fu Y, Appl. Therm. Eng., 149, 1069 (2018)
Pan J, Li R, Lv T, Wu G, Deng Z, Appl. Therm. Eng., 93, 27 (2016)
Qi C, Yi C, Wang B, Wang W, Xu J, Appl. Therm. Eng., 150, 61 (2019)
Singh AK, Tyagi B, Kumar V, Chem. Prod. Process. Model., 8(1), 53 (2013)
Gupta A, CEP, Jun, 22 (2018).
Venkatasubramanian V, AIChE J., 65(2), 466 (2018)
Zhang Z, Wu Z, Rincon D, Christofides PD, Mathematics, 7(10), 890 (2019)
D'alessandro AA, Izurieta EM, Tonelli SM, J. Loss Prev. Process Ind., 43, 255 (2016)
Houde S, Shurong Y, Jianling F, Xing W, Polish Marit. Res., 24(S2), 14 (2017)
Kim H, Park M, Kim CW, Shin D, Comput. Chem. Eng., 125, 476 (2019)
Jin H, Song Q, Hu X, KDD’19, Aug, Anchorage, AK, USA(2019).
Sola J, Sevilla J, IEEE Trans Nucl Sci, 44(3), 1464 (1997)
Wainwright R, Shenfield A, Athens J. Sci., 6(1), 19 (2019)
Kim NK, Yun SK, J. KIGAS, 23(1), 41 (2019)
Su H, Yu S, Fan J, Ling X, J. Comput., 9(2) (2014)
Wang M, Jin T, Tang K, Chen G, Natural Gas Industry, 33, 102 (2013)
Jin T, Wang M, Tang K, Cryogenics, 61, 127 (2014)
Deng Z, Hui K, Zhang Y, Cao Y, Appl. Therm. Eng., 106, 721 (2016)
Cheng H, Ju Y, Fu Y, Appl. Therm. Eng., 149, 1069 (2018)
Pan J, Li R, Lv T, Wu G, Deng Z, Appl. Therm. Eng., 93, 27 (2016)
Qi C, Yi C, Wang B, Wang W, Xu J, Appl. Therm. Eng., 150, 61 (2019)
Singh AK, Tyagi B, Kumar V, Chem. Prod. Process. Model., 8(1), 53 (2013)
Gupta A, CEP, Jun, 22 (2018).
Venkatasubramanian V, AIChE J., 65(2), 466 (2018)
Zhang Z, Wu Z, Rincon D, Christofides PD, Mathematics, 7(10), 890 (2019)
D'alessandro AA, Izurieta EM, Tonelli SM, J. Loss Prev. Process Ind., 43, 255 (2016)
Houde S, Shurong Y, Jianling F, Xing W, Polish Marit. Res., 24(S2), 14 (2017)
Kim H, Park M, Kim CW, Shin D, Comput. Chem. Eng., 125, 476 (2019)
Jin H, Song Q, Hu X, KDD’19, Aug, Anchorage, AK, USA(2019).
Sola J, Sevilla J, IEEE Trans Nucl Sci, 44(3), 1464 (1997)
Wainwright R, Shenfield A, Athens J. Sci., 6(1), 19 (2019)
Kim NK, Yun SK, J. KIGAS, 23(1), 41 (2019)