Overall
- Language
- korean
- Conflict of Interest
- In relation to this article, we declare that there is no conflict of interest.
- Publication history
-
Received August 23, 2024
Revised December 11, 2024
Accepted December 11, 2024
Available online February 1, 2025
-
This is an Open-Access article distributed under the terms of the Creative Commons Attribution Non-Commercial License (http://creativecommons.org/licenses/bync/3.0) which permits unrestricted non-commercial use, distribution, and reproduction in any medium, provided the original work is properly cited.
Most Cited
강화학습을 이용한 천연가스 액화 공정 최적화에 관한 연구
A Study on Optimization of Natural Gas Liquefaction Process Using Reinforcement Learning
https://doi.org/10.9713/kcer.2025.63.1.50
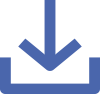
Abstract
본 연구에서는 강화학습 방법론 중 Deep Q-Network(DQN)와 Advantage Actor-Critic(A2C)알고리즘을 이용하여 천
연가스 액화공정 중 단일혼합냉매 공정을 최적화하고 각 알고리즘에 따른 에너지 소모량 결과를 유전 알고리즘(Genetic
algorithm)을 통한 최적화 결과와 비교 분석하였다. 그 결과 DQN 최적화 결과가 A2C보다 낮은 에너지 소모량을 보였
으며 학습 시간은 A2C 알고리즘이 짧은 것을 확인하였다. 그러나 유전 알고리즘과 비교분석 결과 유전 알고리즘의 최
적화 결과가 가장 좋았으며, 강화학습을 통한 공정의 최적화를 위해 연속적인 변수를 다루는 행동 지정에 대한 연구가
필요함을 제시하였다.
In this study, Deep Q-Network and Advantage Actor-Critic algorithms among reinforcement learning
methodologies were used to optimize the single-mixed refrigerant process for a natural gas liquefaction. And optimization
results using these algorithms were compared with the results of genetic algorithm (GA). The results showed that the
optimization results using the DQN algorithm had lower energy consumption than A2C, and the learning time was
shorter for the A2C algorithm. However, the comparison analysis with the genetic algorithm (GA) showed that the GA
had the best performance, suggesting that research on specifying actions that deal with continuous variables is necessary
for optimizing the process through reinforcement learning.
References
2. He, T., Chong, Z. R., Zheng, J., Ju, Y. and Linga, P., “LNG Cold
Energy Utilization: Prospects and Challenges,” Energy, 170, 557-
568(2019).
3. He, T. and Ju, Y., “Optimal Synthesis of Expansion Liquefaction
Cycle for Distributed-Scale LNG (Liquefied Natural Gas)
Plant,” Energy, 88, 268-280(2015).
4. Aspelund, A., Gundersen, T., Myklebust, J., Nowak, M. P. and
Tomasgard, A., “An Optimization-Simulation Model for a Simple
LNG Process,” Computers & Chemical Engineering, 34(10), 1606-
1617(2010).
5. Silver, D., Huang, A., Maddison., C. J., Guez, A., Sifre, L., van
den Driessche, G., Schrittwieser, J., Antonoglou, L., Panneershelvam,
V., Lanctot, M., Dieleman, S., Grewe, D., Nham, J., Kalchnrenner,
N., Sutskever, I., Lilicrap, T., Leach, M., Kavukcuoglu, K.,
Graepel, T. and Hassabis, D., “Mastering the Game of Go with Deep
Neural Networks and Tree Search,” Nature, 529, 484-489(2016).
6. https://www.yokogawa.com/news/press-releases/2022/2022-03-
22/, accessed: 26. Oct. 24.
7. Lee, S. H., Lim, D.-H. and Park, K., “Optimization and Economic
Analysis for Small-Scale Movable LNG Liquefaction Process
with Leakage Considerations,” Appl. Sci., 10(15), 5391(2020).
8. Gao, Q. and Schweidtmann, A. M., “Deep Reinforcement Learning
for Process Design: Review and Perspective,” Current Opinion
in Chemical Engineering, 44, 101012(2024).
9. Kim, S., Jang, M.-G. and Kim, J.-K., “Process Design and Optimization
of Single Mixed-Refrigerant Processes with the Application
of Deep Reinforcement Learning,” Applied Thermal Engineering,
223, 120038(2023).
10. Seidenberg, J. R., Khan, A. A. and Lapkin, A. A., “Boosting
Autonomous Process Design and Intensification with Formalized
Domain Knowledge,” Computers and Chemical Engineering,
169, 108097(2023).
11. Stops, L., Leenhouts, R., Gao, Q. and Schweidtmann, A. M., “Flowsheet
Generation through Hierarchical Reinforcement Learning
and Graph Neural Networks,” AlChe J, 69(1), 17938(2023).
12. Chen, J. and Wang, F., “Cost Reduction of CO2 Capture Processes
Using Reinforcement Learning Based Iterative Design: A Pilot-Scale
Absorption–Stripping System,” Separation and Purification Technology,
122, 149-158(2014).
13. Roh, E. J., Lee, H., Park, S., Kim, J., Kim, K. and Kim, S., “Directional
Autonomous Torpedo Maneuver Control Using Reinforcement
Learning,” The Journal of Korean Institute of Communications
and Information Sciences, 49(5), 752-761(2024).
14. Mnih, V., Kavukcuoglu, K., Silver, D., Rusu, A. A., Veness, J.,
Bellemare, M. G., Graves, A., Riedmiller, M., Fidjeland, A. K.,
Ostrovski, G., Petersen, S., Beattie, C., Sadik, A., Antonoglou, I.,
King, H., Kumaran, D., Wierstra, D., Legg, S. and Hassabis, D.,
“Human-Level Control through Deep Reinforcement Learning,”
Nature, 518(7540), 529-533(2015).
15. Bao, J., Lin, Y., Zhang, R., Zhang, N. and He, G., “Effects of Stage
Number of Condensing Process on the Power Generation Systems
for LNG Cold Energy Recovery,” Applied Thermal Engineering,
126, 566-582(2017).
16. https://pygad.readthedocs.io/en/latest/, accessed: 26. Oct. 24.