Overall
- Language
- korean
- Conflict of Interest
- In relation to this article, we declare that there is no conflict of interest.
- Publication history
-
Received May 30, 2022
Accepted July 14, 2022
-
This is an Open-Access article distributed under the terms of the Creative Commons Attribution Non-Commercial License (http://creativecommons.org/licenses/bync/3.0) which permits unrestricted non-commercial use, distribution, and reproduction in any medium, provided the original work is properly cited.
Copyright © KIChE. All rights reserved.
Most Downloaded
토양에 살포된 축산 분뇨로부터 암모니아 방출량 예측을 위한 인공신경망의 초매개변수 최적화와 데이터 증식
Hyperparameter Optimization and Data Augmentation of Artificial Neural Networks for Prediction of Ammonia Emission Amount from Field-applied Manure
한경대학교 화학공학과 CoSPE 연구센터, 17579 경기도 안성시 중앙로 327
Dept. Chemical Engineering, CoSPE, Hankyong National University, Anseong, 17579, Korea
Korean Chemical Engineering Research, February 2023, 61(1), 123-141(19), 10.9713/kcer.2023.61.1.123 Epub 26 January 2023
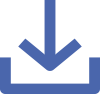
Abstract
인공신경망을 이용한 모델 개발에서 데이터의 품질은 모델 성능에 큰 영향을 주고, 양질의 충분한 데이터가 인공신 경망 훈련을 위해 필요하다. 하지만, 공학 분야에서는 적은 양의 데이터로 모델을 개발해야 하는 경우가 자주 발생한 다. 본 논문은 토양에 살포된 축산 분뇨로부터 암모니아 방출량에 대한 적은 수의 데이터(83개)를 사용하여 인공신경 망 모델의 예측 성능을 향상할 수 있는 방안을 제시하였다. Michaelis-Menten 식으로 표현되는 암모니아 방출량 문제는 11개 입력변수에 대하여 2개 출력변수로 구성되었다. 출력변수는 최대 질소 발생량(Nmax, kg/ha)과 Nmax의 절반에 도달 하는 시간(Km, h)이다. 범주형 입력변수에 대해 다차원 등간격 기법인 one-hot encoding을 이용하여 데이터 전처리를 수행하였고, 훈련데이터 66개에 대하여 generative adversarial network (GAN)을 이용하여 13개 데이터를 추가로 보강 하였다. 또한, 인공신경망의 초매개변수인 은닉층 수, 각 은닉층 내 뉴런 수, 활성화 함수의 최적 조합을 찾기 위하여 Gaussian process (GP)를 사용하였다. 기존의 인공신경망 구조(Lim et al., 2007) 는 17개 평가데이터에 대하여 mean absolute error (MAE)는 Km에서 0.0668, Nmax에서 0.1860이었다. 본 연구에서 제시된 인공신경망 모델은 Km에서 0.0414, Nmax에서 0.0818로 MAE 가 기존 모델 대비 각각 38%, 56% 감소하였다. 본 연구에서 제시된 방법은 적은 양의 데이 터를 갖는 문제에서 인공신경망 성능을 향상하기 위하여 활용할 수 있을 것이다.
A sufficient amount of data with quality is needed for training artificial neural networks (ANNs). However, developing ANN models with a small amount of data often appears in engineering fields. This paper presented an ANN model to improve prediction performance of the ammonia emission amount with 83 data. The ammonia emission rate included eleven inputs and two outputs (maximum ammonia loss, Nmax and time to reach half of Nmax, Km). Categorical input variables were transformed into multi-dimensional equal-distance variables, and 13 data were added into 66 training data using a generative adversarial network. Hyperparameters (number of layers, number of neurons, and activation function) of ANN were optimized using Gaussian process. Using 17 test data, the previous ANN model (Lim et al., 2007) showed the mean absolute error (MAE) of Km and Nmax to 0.0668 and 0.1860, respectively. The present ANN outperformed the previous model, reducing MAE by 38% and 56%.
Keywords
References
Lim Y, Moon YS, Kim TW, Eur. J. Agron., 26(4), 425 (2007)
Sintermann J, Neftel A, Ammann C, Häni C, Hensen A, Loubet B, Flechard CR, Biogeosciences, 9(5), 1611 (2012)
Pedersen J, Andersson K, Feilberg A, Delin S, Hafner S, Nyord T, Biosyst. Eng., 202, 66 (2021)
Moon YS, Lim Y, Kim TW, Korean Chem. Eng. Res., 45(2), 133 (2007)
Jain S, Shukla S, Wadhvani R, Expert Syst. Appl., 106, 252 (2018)
Jackson JE, A User’s Guide to Principal Components, 1st ed., John Wiley and Sons, New York(1991).
Ren J, Chen J, Shi D, Li Y, Li D, Wang Y, Cai D, Int. J. Electr. Power Energy Syst., 136, 107651 (2022)
Hovden IT, “Optimizing Artificial Neural Network Hyperparameters and Architecture,” (2019).
Islam A, Belhaouari SB, Rehman AU, Bensmail H, Appl. Soft Comput., 115, 108288 (2022)
Ngo SI, Lim YI, Catalysts, 11(11), 1304 (2021)
Raissi M, Perdikaris P, Karniadakis GE, J. Comput. Phys., 378, 686 (2019)
Yang Y, Zha K, Chen YC, Wang H, Katabi D, “Delving into Deep Imbalanced Regression,” (2021).
Zhuo Y, Brgoch J, J. Phys. Chem. Lett., 12(2), 764 (2021)
Coomans D, Massart DL, Anal. Chim. Acta, 136, 15 (1982)
Chawla NV, Bowyer KW, Hall LO, Kegelmeyer WP, J. Artif. Intell. Res., 16, 321 (2002)
Torgo L, Ribeiro R, Pfahringer B, Branco P, Smote for Regression, ed., Springer, Berlin, Heidelberg, Correia(2013).
Goodfellow I, Pouget-Abadie J, Mirza M, Xu B, Warde-Farley D, Ozair S, Courville A, Bengio Y, Adv. Neural Inf. Process. Syst., 27 (2014)
Asadi M, McPhedran KN, Sci. Total Environ., 800, 149508 (2021)
Du X, Xu H, Zhu F, Comput. Aided Des, 135, 103013 (2021)
Bergstra J, Bardenet R, Bengio Y, Kégl B, Adv. Neural Inf. Process. Syst., (2011).
Yang Z, Zhang A, J. Mach. Learn. Res., 22(149), 1 (2021)
Yang L, Shami A, Neurocomputing, 415, 295 (2020)
Jankovic A, Chaudhary G, Goia F, Energy Build., 250, 111298 (2021)
Misselbrook TH, Nicholson FA, Chambers BJ, Bioresour. Technol., 96(2), 159 (2005)
Rodríguez P, Bautista MA, Gonzàlez J, Escalera S, Image Vis. Comput., 75, 21 (2018)
Demir S, Mincev K, Kok K, Paterakis NG, Appl. Energy, 304, 117695 (2021)
Ramachandran P, Zoph B, Le QV, “Searching for Activation Functions,” arXiv preprint arXiv:1710.05941, (2017).
LeCun Y, Bengio Y, Hinton G, Nature, 521(7553), 436 (2015)
Kohavi R, “A Study of Cross-Validation and Bootstrap for Accuracy Estimation and Model Selection,” pp. 1137-1143, (1995).
li W, Liu Z, Procedia Environ. Sci., 11, 256 (2011)
Jahirul MI, Rasul MG, Brown RJ, Senadeera W, Hosen MA, Haque R, Saha SC, Mahlia TMI, Renew. Energy, 168, 632 (2021)
Liu Y, Zhao S, Wang Q, Gao Q, Neurocomputing, 275, 924 (2018)
Berk J, Nguyen V, Gupta S, Rana S, Venkatesh S, “Exploration Enhanced Expected Improvement for Bayesian Optimization, Cham(2018).
Riedmiller M, Braun H, “A Direct Adaptive Method for Faster Backpropagation Learning: The Rprop Algorithm,” IEEE International Conference on Neural Networks, March, 1993.
Sintermann J, Neftel A, Ammann C, Häni C, Hensen A, Loubet B, Flechard CR, Biogeosciences, 9(5), 1611 (2012)
Pedersen J, Andersson K, Feilberg A, Delin S, Hafner S, Nyord T, Biosyst. Eng., 202, 66 (2021)
Moon YS, Lim Y, Kim TW, Korean Chem. Eng. Res., 45(2), 133 (2007)
Jain S, Shukla S, Wadhvani R, Expert Syst. Appl., 106, 252 (2018)
Jackson JE, A User’s Guide to Principal Components, 1st ed., John Wiley and Sons, New York(1991).
Ren J, Chen J, Shi D, Li Y, Li D, Wang Y, Cai D, Int. J. Electr. Power Energy Syst., 136, 107651 (2022)
Hovden IT, “Optimizing Artificial Neural Network Hyperparameters and Architecture,” (2019).
Islam A, Belhaouari SB, Rehman AU, Bensmail H, Appl. Soft Comput., 115, 108288 (2022)
Ngo SI, Lim YI, Catalysts, 11(11), 1304 (2021)
Raissi M, Perdikaris P, Karniadakis GE, J. Comput. Phys., 378, 686 (2019)
Yang Y, Zha K, Chen YC, Wang H, Katabi D, “Delving into Deep Imbalanced Regression,” (2021).
Zhuo Y, Brgoch J, J. Phys. Chem. Lett., 12(2), 764 (2021)
Coomans D, Massart DL, Anal. Chim. Acta, 136, 15 (1982)
Chawla NV, Bowyer KW, Hall LO, Kegelmeyer WP, J. Artif. Intell. Res., 16, 321 (2002)
Torgo L, Ribeiro R, Pfahringer B, Branco P, Smote for Regression, ed., Springer, Berlin, Heidelberg, Correia(2013).
Goodfellow I, Pouget-Abadie J, Mirza M, Xu B, Warde-Farley D, Ozair S, Courville A, Bengio Y, Adv. Neural Inf. Process. Syst., 27 (2014)
Asadi M, McPhedran KN, Sci. Total Environ., 800, 149508 (2021)
Du X, Xu H, Zhu F, Comput. Aided Des, 135, 103013 (2021)
Bergstra J, Bardenet R, Bengio Y, Kégl B, Adv. Neural Inf. Process. Syst., (2011).
Yang Z, Zhang A, J. Mach. Learn. Res., 22(149), 1 (2021)
Yang L, Shami A, Neurocomputing, 415, 295 (2020)
Jankovic A, Chaudhary G, Goia F, Energy Build., 250, 111298 (2021)
Misselbrook TH, Nicholson FA, Chambers BJ, Bioresour. Technol., 96(2), 159 (2005)
Rodríguez P, Bautista MA, Gonzàlez J, Escalera S, Image Vis. Comput., 75, 21 (2018)
Demir S, Mincev K, Kok K, Paterakis NG, Appl. Energy, 304, 117695 (2021)
Ramachandran P, Zoph B, Le QV, “Searching for Activation Functions,” arXiv preprint arXiv:1710.05941, (2017).
LeCun Y, Bengio Y, Hinton G, Nature, 521(7553), 436 (2015)
Kohavi R, “A Study of Cross-Validation and Bootstrap for Accuracy Estimation and Model Selection,” pp. 1137-1143, (1995).
li W, Liu Z, Procedia Environ. Sci., 11, 256 (2011)
Jahirul MI, Rasul MG, Brown RJ, Senadeera W, Hosen MA, Haque R, Saha SC, Mahlia TMI, Renew. Energy, 168, 632 (2021)
Liu Y, Zhao S, Wang Q, Gao Q, Neurocomputing, 275, 924 (2018)
Berk J, Nguyen V, Gupta S, Rana S, Venkatesh S, “Exploration Enhanced Expected Improvement for Bayesian Optimization, Cham(2018).
Riedmiller M, Braun H, “A Direct Adaptive Method for Faster Backpropagation Learning: The Rprop Algorithm,” IEEE International Conference on Neural Networks, March, 1993.