Articles & Issues
- Language
- english
- Conflict of Interest
- In relation to this article, we declare that there is no conflict of interest.
- Publication history
-
Received May 14, 2022
Revised August 26, 2022
Accepted December 16, 2022
-
This is an Open-Access article distributed under the terms of the Creative Commons Attribution Non-Commercial License (http://creativecommons.org/licenses/bync/3.0) which permits unrestricted non-commercial use, distribution, and reproduction in any medium, provided the original work is properly cited.
All issues
Performance of Cu-SiO2 Aerogel Catalyst in Methanol Steam Reforming: Modeling of hydrogen production using Response Surface Methodology and Artificial Neuron Networks
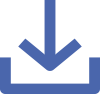
Abstract
Methanol steam reforming (MSR) is a promising method for hydrogen supplying as a critical step in
hydrogen fuel cell commercialization in mobile applications. Modelling and understanding of the reactor behavior is an
attractive research field to develop an efficient reformer. Three-layer feed-forward artificial neural network (ANN) and
Box-Behnken design (BBD) were used to modelling of MSR process using the Cu-SiO2 aerogel catalyst. Furthermore,
impacts of the basic operational variables and their mutual interactions were studied. The results showed that the most
affecting parameters were the reaction temperature (56%) and its quadratic term (20.5%). In addition, it was also found
that the interaction between temperature and Steam/Methanol ratio is important on the MSR performance. These models
precisely predict MSR performance and have great agreement with experimental results. However, on the basis of
statistical criteria the ANN technique showed the greater modelling ability as compared with statistical BBD approach.
Keywords
References
2. Ke, Y., Zhou, W., Chu, X., Yuan, D., Wan, S., Yu, W. and Liu,Y., “Porous Copper Fiber Sintered Felts with Surface Microchannels for Methanol Steam Reforming Microreactor for Hydrogen Production,” International J. Hydrogen Energy, 44(12), 5755-5765(2019).
3. Liu, Y., Zhou, W., Chen, L., Lin, Y., Xuyang, C., Zheng, T. and Wan, S., “Optimal Design and Fabrication of Surface Microchannels on Copper Foam Catalyst Support in a Methanol Steam Reforming Microreactor,” Fuel, 253, 1545-1555(2019).
4. Ouyang, K., Wu, H. W., Huang, S. C. and Wu, S. J., “Optimum Parameter Design for Performance of Methanol Steam Reformer Combining Taguchi Method with Artificial Neural Network and Genetic Algorithm,” Energy, 138, 446-458(2017).
5. Perng, S.-W., Horng, R.-F. and Ku, H.-W., “Numerical Predictions of Design and Operating Parameters of Reformer on the Fuel Conversion and CO Production for the Steam Reforming of Methanol,” International J. Hydrogen Energy, 38(2), 840-852(2013).
6. Tahay, P., Khani, Y., Jabari, M., Bahadoran, F., Safari, N. and Zamanian, A., “Synthesis of Cubic and Hexagonal ZnTiO3 as Catalyst Support in Steam Reforming of Methanol: Study of Physical and Chemical Properties of Copper Catalysts on the H2
and CO Selectivity and Coke Formation,” International J. Hydrogen Energy (2020).
7. Fasanya, O. O., et al., “Copper Zinc Oxide Nanocatalysts Grown on Cordierite Substrate for Hydrogen Production Using Methanol Steam Reforming,” International J. Hydrogen Energy, 44(41),22936-22946 (2019).
8. Phongboonchoo, Y., Thouchprasitchai, N. and Pongstabodee, S.,“Hydrogen Production with a Low Carbon Monoxide Content via Methanol Steam Reforming over CuxCeyMgz/Al2O3 Catalysts: Optimization and Stability,” International J. Hydrogen Energy,42(17), 12220-12235(2017).
9. Qing, S.-J., et al., “Catalytic Performance of Cu-Ni-Al Spinel for Methanol Steam Reforming to Hydrogen,” J. Fuel Chemistry and Technology, 46(10), 1210-1217(2018).
10. Sarafraz, M. M., et al., “Reforming of Methanol with Steam in a Micro-reactor with Cu–SiO2 Porous Catalyst,” International J.Hydrogen Energy, 44(36), 19628-19639(2019).
11. Ribeirinha, P., et al., “Study of Different Designs of Methanol Steam Reformers: Experiment and Modeling,” International J.Hydrogen Energy, 39(35), 19970-19981(2014).
12. Shanmugam, V., et al., “Hydrogen Production over Highly Active Pt Based Catalyst Coatings by Steam Reforming of Methanol:Effect of Support and co-support,” International J. Hydrogen Energy,45(3), 1658-1670(2020).
13. Tang, H.-Y., Greenwood, J. and Erickson, P., “Modeling of a Fixed-bed Copper-based Catalyst for Reforming Methanol: Steam and Autothermal Reformation,” International J. Hydrogen Energy,40(25), 8034-8050(2015).
14. Herdem, M. S., Farhad, S. and Hamdullahpur, F., “Modeling and Parametric Study of a Methanol Reformate Gas-fueled HTPEMFC System for Portable Power Generation Applications,”Energy Conversion and Management, 101, 19-29(2015).
15. Wan, Y., Zhou, Z. and Cheng, Z., “Hydrogen Production from Steam Reforming of Methanol over CuO/ZnO/Al2O3 Catalysts:Catalytic Performance and Kinetic Modeling,” Chinese J. Chemical Engineering, 24(9), 1186-1194(2016).
16. Vidal Vázquez, F., et al., “Reactor Design and Catalysts Testing for Hydrogen Production by Methanol Steam Reforming for Fuel
Cells Applications,” International J. Hydrogen Energy, 41(2),924-935(2016).
17. Wu, W., et al., “Design, Modeling, and Optimization of a Lightweight MeOH-to-H2 Processor,” International J. Hydrogen Energy,
43(31), 14451-14465(2018).
18. Sari, A. and Sabziani, J., “Modeling and 3D-simulation of Hydrogen Production via Methanol Steam Reforming in Copper-coated
Channels of a Mini Reformer,” J. Power Sources, 352, 64-76(2017).
19. He, J., et al., “Cu Supported on ZnAl-LDHs Precursor Prepared by in-situ synthesis method on γ-Al2O3 as Catalytic Material with High Catalytic Activity for Methanol Steam Reforming,”International J. Hydrogen Energy, 42(15) 9930-9937(2017).
20. Ma, H., et al., “Two-dimensional Modeling of a Plant-scale Fixedbed Reactor for Hydrogen Production from Methanol Steam Reforming,” International J. Hydrogen Energy, 41(38), 16932-16943(2016).
21. Chuang, C.-C., et al., “Optimal Design of An Experimental Methanol Fuel Reformer,” International J. Hydrogen Energy, 33(23),7062-7073(2008).
22. Jang, J.-Y., Huang, Y.-X. and Cheng, C.-H., “The Effects of Geometric and Operating Conditions on the Hydrogen Production Performance of a Micro-methanol Steam Reformer,” Chemical Engineering Science, 65(20), 5495-5506(2010).
23. Zheng, T., et al., “Methanol Steam Reforming Performance Optimisation of Cylindrical Microreactor for Hydrogen Production Utilising Error Backpropagation and Genetic Algorithm,” Chemical Engineering J., 357, 641-654(2019).
24. Purnama, H., Catalytic Study of Copper based Catalysts for Steam Reforming of Methanol, Ph.D. Dissertation, Technical University of Berlin, Berlin (2003).
25. Matsumura, Y. and H. Ishibe, “Selective Steam Reforming of Methanol over Silica-supported Copper Catalyst Prepared by Sol–gel Method,” Applied Catalysis B: Environmental, 86(3),114-120(2009).
26. Takezawa, N., et al., “Steam Reforming of Methanol on Coppersilica Catalysts; Effect of Copper Loading and Calcination Temperature on the Reaction,” Applied Catalysis, 4(2), 127-134(1982).
27. Takezawa, N. and N. Iwasa, “Steam Reforming and Dehydrogenation of Methanol: Difference in the Catalytic Functions of Copper and Group VIII Metals,” Catalysis Today, 36(1), 45-56(1997).
28. Tajrishi, O.Z., M. Taghizadeh, and A.D. Kiadehi, “Methanol Steam Reforming in a Microchannel Reactor by Zn-, Ce- and ZrModified Mesoporous Cu/SBA-15 Nanocatalyst,” International J. Hydrogen Energy, 43(31), 14103-14120(2018).
29. Yousefi Amiri, T. and J. Moghaddas, “Cogeled Copper–silica Aerogel as a Catalyst in Hydrogen Production from Methanol
Steam Reforming,” International J. Hydrogen Energy, 40(3), 1472-1480(2015).
30. Amiri, T. Y. and Moghaddas, J., “Reaction Parameters Influence on the Catalytic Performance of Copper-silica Aerogel in the Methanol Steam Reforming,” J. Fuel Chemistry and Technology,44(1), 84-90(2016).
31. Yousefi Amiri, T. and Moghaddas, J., “Performance Evaluation of Cu-SiO2 Aerogel Catalyst in Methanol Steam Reforming,”Iranian J. Chemical Engineering(IJChE), 11(3), 37-44 (2014).
32. Yousefi Amiri, T., Moghaddas, J. and Rahmani Khajeh, S., “Silica Aerogel-supported Copper Catalyst Prepared Via Ambient Pressure Drying Process,” J. Sol-Gel Science and Technology,77(3), 627-635(2016).
33. Chiu, Y.-J., et al., “Simulations of Hydrogen Production by Methanol Steam Reforming,” Energy Procedia, 156, 38-42(2019).
34. Kakelar, M. and S. Ebrahimi, “Up-scaling Application of Microbial Carbonate Precipitation: Optimization of Urease Production
Using Response Surface Methodology and Injection Modification,” International J. Environmental Science and Technology,13(11), 2619-2628(2016).
35. Karthic, P., et al., “Optimization of Biohydrogen Production by Enterobacter Species Using Artificial Neural Network and Response
Surface Methodology,” J. Renewable and Sustainable Energy,5(3), 033104(2013).
36. Chiu, Y.-J., et al., “Experimental Study on the Reaction Conditions of a Methanol Steam Reforming Process,” Energy Procedia, 105, 1622-1627(2017).
37. Liu, C.-H., Hwang, C.-F. and Liao, C.-C., “Medium Optimization for Glutathione Production by Saccharomyces Cerevisiae,” Process Biochemistry, 34(1), 17-23(1999).
38. He, L., Xu, Y. Q. and Zhang, X. H., “Medium Factor Optimization and Fermentation Kinetics for Phenazine-1-carboxylic Acid
Production by Pseudomonas sp. M18G,” Biotechnology and Bioengineering, 100(2), 250-259(2008).
39. Wang, J. and Wan, W., “Optimization of Fermentative Hydrogen Production Process Using Genetic Algorithm Based on Neural
Network and Response Surface Methodology,” International J.Hydrogen Energy, 34(1), 255-261(2009).
40. Nasr, N., et al., “Application of Artificial Neural Networks for Modeling of Biohydrogen Production,” International J. Hydrogen Energy, 38(8), 3189-3195(2013).
41. Adib, H., et al., “Modeling and Optimization of Fischer–Tropsch Synthesis in the Presence of Co (III)/Al2O3 Catalyst Using Artificial Neural Networks and Genetic Algorithm,” J. Natural Gas Science and Engineering, 10, 14-24(2013).
42. Dawson, C. W. and Wilby, R., “An Artificial Neural Network Approach to Rainfall-runoff Modelling,” Hydrological Sciences J., 43(1), 47-66(1998).
43. Aghaeinejad-Meybodi, A., et al., “Modeling and Optimization of Antidepressant Drug Fluoxetine Removal in Aqueous Media
by Ozone/H2O2 Process: Comparison of Central Composite Design and Artificial Neural Network Approaches,” J. Taiwan Institute
Chemical Engineers, 48, 40-48(2015).
44. Asadzadeh, F., Maleki-Kakelar, M. and Shabani, F., “Predicting Cationic Exchange Capacity in Calcareous Soils of East-Azerbaijan Province, Northwest Iran,” Communications in Soil Science and Plant Analysis, 50(9), 1106-1116(2019).
45. Salehi, E., et al., “Data-based Modeling and Optimization of a Hybrid Column-adsorption/depth-filtration Process Using a Combined Intelligent Approach,” J. Cleaner Production, 236, 117664(2019).
46. Khataee, A. R. and Kasiri, M. B., “Artificial Neural Networks Modeling of Contaminated Water Treatment Processes by Homogeneous and Heterogeneous Nanocatalysis,” J. Molecular Catalysis A: Chemical, 331(1), 86-100(2010).
47. Kumar, N. M., Ramasamy, R., and Manonmani, H., “Production and Optimization of l-asparaginase from Cladosporium sp.
Using Agricultural Residues in Solid State Fermentation,” Indus-trial Crops and Products, 43, 150-158(2013).
48. Maddipati, P., et al., “Ethanol Production from Syngas by Clostridium Strain P11 Using Corn Steep Liquor as a Nutrient Replacement to Yeast Extract,” Bioresource Technology, 102(11), 6494-6501(2011).
49. Aghaeinejad-Meybodi, A., et al., “Degradation of Fluoxetine Using Catalytic Ozonation in Aqueous Media in the Presence of Nano-γ-alumina Catalyst: Experimental, Modeling and Optimization Study,” Separation and Purification Technology, 211, 551-563(2019).
50. Aghaeinejad-Meybodi, A., et al., “Degradation of Antidepressant Drug Fluoxetine in Aqueous Media by Ozone/H2O2 System:
Process Optimization Using Central Composite Design,” Environmental Technology, 36(12), 1477-1488(2015).
51. Baş, D. and Boyacı, İ. H., “Modeling and Optimization II: Comparison of Estimation Capabilities of Response Surface Methodology with Artificial Neural Networks in a Biochemical Reaction,”J. Food Engineering, 78(3), 846-854(2007).
52. Ghafari, E., Costa, H. and Júlio, E., “RSM-based Model to Predict the Performance of Self-compacting UHPC Reinforced with
Hybrid Steel Micro-fibers,” Construction and Building Materials, 66, 375-383(2014).